Optimal Experiment Design
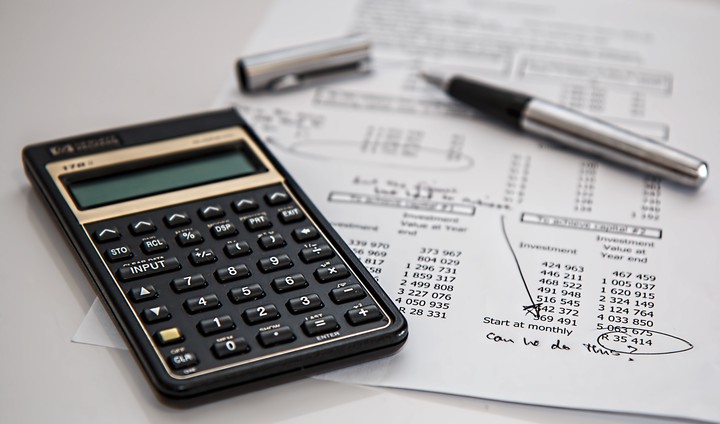
Introduction
When planning an A/B test, computing the statistical power is an excellent way to decide how large a sample size we need to achieve the desired sensitivity. When analyzing a test, computing a confidence interval on the parameter(s) of interest gives us a range of possibilities consistent with the data. We can connect these ideas using the techniques of Optimal Experiment Design: we can choose the sample size to achieve a desired confidence interval width!
Let’s look at an example from a linear model. Suppose we are planning an experiment with $n$ experimental units, which will be randomly segmented into $m$ experiment groups. Different experiences will be provided to each group. The experience provided to group $i$ is encoded by a vector $\nu_i \in \mathbb{R}^p$, $i=1,\ldots,m$. Suppose the $j$th experimental unit is assigned to group $i_j$, and let $V \in \mathbb{R}^{n \times p}$ be the matrix whose $j$th row is $\nu_{i_j}$. The response $y$ is assumed to be normally distributed with mean $V \beta$ and covariance $\sigma^2 I$. An elementary result in linear regression is that the maximum likelihood estimate of $\beta$ is $\hat{\beta} = (V^T V)^{-1} V^T y$. We will assume that $\sigma^2$ is unknown and must be estimated from the data. An unbiased estimator is $\hat{\sigma}^2 = \| y - V \hat{\beta} \|_2^2 / (n - p)$.
A $100(1-\alpha)\%$ confidence interval on $c^T \beta$ has endpoints $$ c^T \hat{\beta} \pm t_{n-p}^{1-\alpha/2} \cdot \sqrt{c^T \hat{\mathcal{I}}^{-1} c}, $$ where $t_{n-p}^{1-\alpha/2}$ is the upper $\alpha/2$ quantile of a Student’s $t$ distribution with $n-p$ degrees of freedom, and $\hat{\mathcal{I}} = V^T V / \hat{\sigma}^2$ is the estimated Fisher information.
For example, when $c = \nu_i - \nu_j$, this is a confidence interval on the difference in mean response between experiment groups $i$ and $j$. Or when $c = \hat{e}_k$, this is a confidence interval on $\beta_k$.
The width of the confidence interval is simply $2 \cdot t_{n-p}^{1-\alpha/2} \cdot \sqrt{c^T \hat{\mathcal{I}}^{-1} c}$. The widest the interval could be (assuming $\| c \|_2 = 1$) is when $c$ is the eigenvector corresponding to the largest eigenvalue of $\hat{\mathcal{I}}^{-1}$, or equivalently, when $c$ is the eigenvector corresponding to the smallest eigenvalue of $\hat{\mathcal{I}}$. Call this minimum eigenvalue $\lambda_\textrm{min}$. In that case, the width of the confidence interval will be $2 \cdot t_{n-p}^{1-\alpha/2} / \sqrt{\lambda_\textrm{min}}$.
Suppose we want to design an experiment so that this width will be less than $h$. Then we need $$ \lambda_\textrm{min} \geq 4 \left( \frac{t_{n-p}^{1-\alpha/2}}{h} \right)^2. $$
Since $V^T V = \sum_{j=1}^n \nu_{i_j} \nu_{i_j}^T$, if $n_i$ units are assigned to experiment group $i$, then $V^T V = \sum_{i=1}^m n_i \nu_i \nu_i^T$. We arrive at the optimization problem $$ \begin{array}{ll} \mbox{minimize} & 1^T w \\\ \mbox{subject to} & \lambda_\textrm{min}(\sum_{i=1}^m w_i \nu_i \nu_i^T) \geq q \\\ & w \succeq 0 \\\ & w_i , \textrm{integers}, \end{array} $$ where $q = 4 \hat{\sigma}^2 (t_{n-p}^{1-\alpha/2} / h)^2$. Of course, to calculate $q$ we need an estimate of $\hat{\sigma}^2$. A reasonably conservative estimate should be used when in doubt, perhaps based on an earlier experiment.
This is a non-convex problem, but if we ignore the integer constraint, the problem is equivalent to: $$ \begin{array}{ll} \mbox{minimize} & 1^T w \\\ \mbox{subject to} & \sum_{i=1}^m w_i \nu_i \nu_i^T - q I \succeq 0 \\\ & w \succeq 0, \end{array} $$ which is a semidefinite program and can be efficiently solved. Rounding the solution, $w$, up to the nearest integers tells us how many experimental units we need in each group. Pretty nifty!
Further Reading
Seber and Lee is my go-to reference on Linear Regression. Boyd and Vandenberghe is a fantastic reference on Convex Optimization, including semidefinite programming and optimal experiment design.